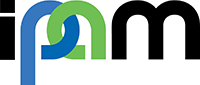
Machine Assisted Proofs
IPAM - July 2023
(By Jordan Ellenberg) In February of 2023, IPAM hosted a workshop on Machine-Assisted Proof. This program had been in the works for more than a year, but ended up being held at a timely moment, when unexpectedly rapid development in large language models (LLMs) in late 2022 had brought focused national attention to the question of how machines could assist or even replace human ingenuity.
As the title of the workshop suggests, the speakers at the conference focused on the former outlook, in which novel techniques in machine learning are potential boosters of human creativity and effort. Our workshop was the first to bring together three groups that have largely worked separately: machine learning developers, often from industry; pure mathematicians at the forefront of using machine learning as a tool in their own work; and formalizers, researchers leading the project of encoding contemporary research mathematics in a form that can be interpreted and checked by machines. The dominant mood at the workshop was one of excitement and synergy. We saw Geordie Williamson (“What Can The Working Mathematician Expect From Deep Learning?” talk about his collaboration with DeepMind, in which machine learning was used to identify a potential conjecture in representation theory which human mathematicians are hard at work on proving; Heather Macbeth (“Algorithm and Abstraction in Formal Mathematics”) talked about the ways our value system as mathematicians will have to change as more mathematics is formalized; and Tony Wu, from Google (“Autoformalization with Large Language Models”) talked about a grand project that synthesized many of the themes of the workshop, namely: using LLMs to generate short formal proofs of desired mathematical statements, which – being formal – can then be automatically checked by the proof verifiers build by the formalization community. In formal mathematics, by contrast with so many other domains where LLMs are being applied, there are robust barriers against “hallucination”!
Participants left the workshop with a feeling of wide-open potential and a clear sense of avenues for future work at the interface between machine learning and pure mathematics.